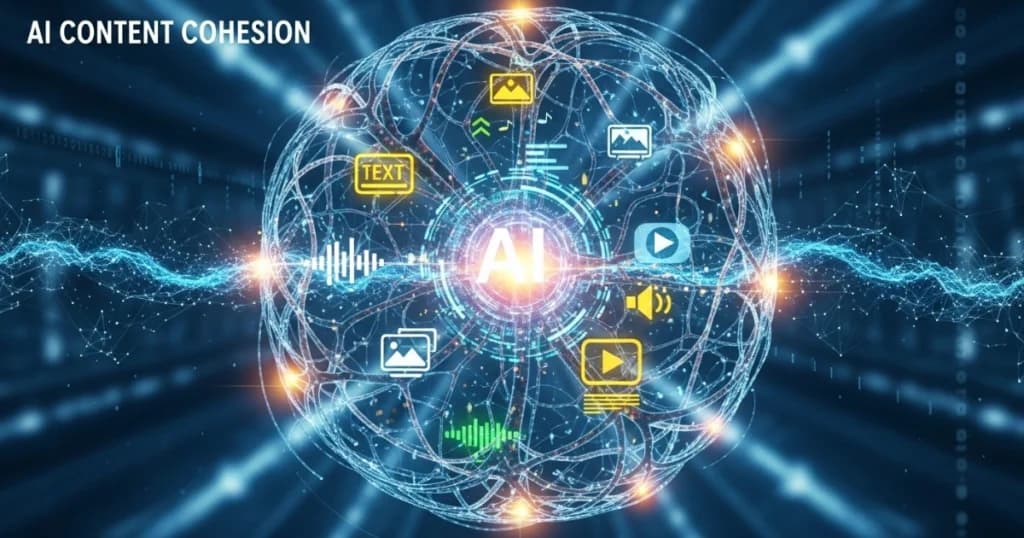
Contents
In today’s era of high-definition displays and 4K streaming, video quality matters more than ever. As a result, AI-powered video upscaling has emerged as a revolutionary technology that enhances the visual clarity of low-resolution videos. By using artificial intelligence, videos that were once grainy or pixelated can now be upscaled to look sharper, smoother, and more lifelike.
This process is powered by deep learning models, which have been trained on vast datasets of high- and low-quality video frames. Consequently, patterns, edges, and missing details are intelligently predicted and reconstructed. Unlike traditional methods, where simple pixel duplication was applied, AI algorithms make context-aware decisions, leading to superior visual improvements.
Furthermore, the adoption of this technology has been accelerated by increased demand for better video quality on modern devices. It is being used in gaming, film restoration, streaming services, and even smartphone apps. As more industries recognize its value, AI-powered video upscaling is expected to play a crucial role in the future of digital media enhancement.
AI-powered video upscaling refers to the process of enhancing the resolution and quality of a video using artificial intelligence. Instead of relying solely on traditional algorithms, this method employs machine learning models trained to recognize and recreate details that may be missing in lower-resolution content.
In conventional upscaling, the image is enlarged by duplicating pixels, which often leads to a blurry or blocky appearance. However, with AI-powered approaches, frames are analyzed, and new pixels are generated based on learned patterns and visual cues. As a result, edges appear sharper, textures become more defined, and motion is rendered more smoothly.
Typically, neural networks especially convolutional neural networks (CNNs) are trained on large sets of high-resolution images. These models learn how real-world objects should look and then apply that knowledge to enhance low-res footage. Once training has been completed, the upscaling model can be applied to videos quickly and efficiently.
Additionally, it should be noted that this technology is not limited to visual enhancement alone. Often, accompanying improvements to color correction, dynamic range, and noise reduction are integrated into the process. Therefore, AI-powered video upscaling delivers a comprehensive upgrade that goes beyond just resolution.
The process behind AI-powered video upscaling is both complex and highly efficient. At its core, it involves using deep learning algorithms to enhance video frames intelligently. Initially, low resolution frames are passed through a neural network that has been trained on thousands or even millions of image and video samples. Consequently, the system learns how to fill in missing details that standard upscaling methods typically cannot reconstruct.
In practice, a convolutional neural network (CNN) is commonly used for this task. These networks excel at image recognition and enhancement because they process spatial information in a way that mimics the human visual system. During training, high-resolution and low-resolution pairs are fed into the system so it can learn the mapping between the two. Once trained, the model can predict what high-resolution content should look like when given a low-res input.
In many cases, additional AI techniques such as generative adversarial networks (GANs) are integrated to add further realism and detail. GANs operate by having two networks compete: one generates upscaled content, while the other evaluates its authenticity. As a result, the output becomes more refined over time.
To optimize performance, the process is often divided into multiple stages. First, noise and compression artifacts are removed. Next, resolution is increased. Finally, contrast, color depth, and motion smoothness are enhanced. All of this happens in real time or near real time, depending on the hardware being used.
Therefore, AI-powered video upscaling not only enlarges video dimensions but also significantly improves quality, making old or low-res content suitable for modern high-definition displays.
Several advanced technologies power the effectiveness of AI-powered video upscaling, allowing it to outperform traditional upscaling methods. By leveraging machine learning and neural network architectures, enhanced resolution and visual quality are achieved in a way that appears both natural and highly detailed.
CNNs are the foundation of most AI upscaling systems. These networks have been designed to detect patterns, edges, and textures in visual data. As a result, they can recognize and reconstruct fine details that are typically lost in low-resolution footage. The upscaling process is automated and improved with each training cycle.
GANs consist of two neural networks: a generator and a discriminator. While the generator creates enhanced images, the discriminator evaluates their authenticity. Over time, the generator learns to produce more realistic frames, improving both the accuracy and visual fidelity of the upscaled content. This approach has been widely adopted in high-end video restoration projects.
Super-resolution refers to the process of reconstructing high-resolution images from low-resolution inputs. It can be performed using single-image super-resolution (SISR) or video-based models. When paired with AI, these algorithms predict and enhance image details beyond the capability of standard mathematical interpolation.
In video upscaling, it is essential to maintain visual coherence across frames. Therefore, temporal consistency modules are used to ensure that objects and motion remain stable from frame to frame. These modules reduce flickering, ghosting, and jittering common artifacts in poorly upscaled videos.
These filters are applied either before or during the upscaling process. They help remove compression artifacts, reduce noise, and sharpen outlines. As a result, the final output appears cleaner and more refined.
By combining these cutting-edge technologies, AI-powered video upscaling delivers results that are not only sharper but also more faithful to the original visual intent. Moreover, these tools continue to evolve, pushing the boundaries of what’s possible in digital video enhancement.
The rise of AI-powered video upscaling has introduced a range of powerful benefits across industries. From content creation to restoration and entertainment, this technology is transforming how visual media is experienced.
One of the most obvious advantages is the significant improvement in image clarity. Low-resolution videos are automatically upscaled to higher resolutions, with sharper edges, more accurate details, and smoother motion. As a result, videos become visually appealing on modern high-resolution displays.
Rather than re-shooting or re-rendering old footage, AI-powered video upscaling allows legacy content to be revived without major production expenses. Archived materials, documentaries, or home videos can be made suitable for current platforms and audiences, saving both time and money.
Streaming platforms, gaming companies, and mobile apps benefit directly from this technology. Viewers enjoy higher-quality visuals without needing ultra-high-bandwidth connections. Since content can be stored in lower resolutions and enhanced in real-time, load times and data usage are reduced while maintaining quality.
Thanks to advances in processing power and algorithm efficiency, AI-based upscaling can now be performed in real-time. Consequently, it is being used in live broadcasts, video calls, and gaming environments to improve visual output instantly.
Whether content is viewed on a smartphone, laptop, or 4K television, AI-powered video upscaling ensures that resolution is optimized for the screen in use. This adaptability leads to consistent quality across all viewing platforms.
Storing videos in lower resolutions and upscaling them when needed reduces file size and energy consumption in data centers. Therefore, it contributes to more sustainable and eco-friendly content delivery systems.
Overall, the implementation of AI-powered video upscaling delivers tangible value not only in terms of aesthetics but also in cost savings, performance, and environmental impact.
The practical use of AI-powered video upscaling has expanded rapidly across various industries. Thanks to its ability to enhance visuals without needing original high-resolution assets, it is being applied in both creative and technical domains.
Classic movies and television shows are often preserved in outdated formats. By using AI-powered video upscaling, these old titles are being digitally restored and re-released in HD or 4K quality. As a result, viewers can enjoy timeless content with modern clarity and detail.
Platforms like YouTube, Netflix, and Amazon Prime benefit from this technology to improve playback quality, especially on slower internet connections. While videos may be streamed in lower resolutions, AI upscaling ensures they appear sharp and smooth on high-resolution displays.
In the world of gaming, performance and visuals are key. Game developers and console manufacturers use AI upscaling to boost frame rates while maintaining visual quality. For instance, a game rendered in 1080p can be upscaled to 4K in real-time without heavily taxing the system’s GPU.
In security systems, footage from older or low-res cameras can be enhanced using AI algorithms. This helps in recognizing faces, license plates, or activities more clearly, making investigations and monitoring far more effective.
Apps that allow video editing and enhancement such as TikTok, Instagram, and CapCut—have started integrating AI-powered upscaling to help users improve their videos with a single click. Therefore, even amateur creators can produce professional-looking content.
In scientific and medical fields, low-resolution video data (like from old scanning equipment or microscopy footage) can be upscaled to reveal finer details, aiding in diagnosis, analysis, and research documentation.
These real-world applications demonstrate how AI-powered video upscaling is not just a trend but a fundamental shift in how video quality is managed and improved across various sectors.
While AI-powered video upscaling offers impressive advantages, it is not without its limitations and challenges. Understanding these drawbacks is essential for evaluating the technology’s practical use and long-term viability.
AI upscaling models require significant processing power, particularly when applied in real time or at higher resolutions. Consequently, high-end GPUs or cloud-based processing environments are often needed. For smaller organizations or independent users, this can lead to increased costs and limited accessibility.
The accuracy of upscaling is heavily reliant on the quality and diversity of the training data. If a model has been trained on a narrow dataset, it may introduce artifacts or unrealistic details when processing unfamiliar content. Therefore, performance may vary across different video genres or visual styles.
AI models sometimes generate overly sharpened or “plastic” textures. While the output might look clearer, it may not always be faithful to the original source material. This over-enhancement can distract viewers and reduce the authenticity of the video, especially in archival or documentary footage.
Various tools and platforms offer AI-powered upscaling, but there is little consensus on best practices or benchmark quality. As a result, outputs can differ dramatically depending on the software or model being used, leading to inconsistent experiences.
When dealing with heavily compressed or damaged videos, AI may struggle to recover accurate details. While it can estimate and fill in missing information, the final result might still fall short of expectations. In such cases, manual restoration methods may still be required.
Altering video content with AI, even for enhancement purposes, raises questions about authenticity and manipulation. Therefore, clear guidelines are needed, especially in journalism, legal evidence, and historical preservation where accuracy must not be compromised.
Although AI-powered video upscaling is transforming visual quality across sectors, its application must be balanced with these technical and ethical considerations. With ongoing research and development, many of these limitations are expected to be addressed in the future.
A clear distinction exists between AI-powered video upscaling and traditional upscaling methods. While both aim to improve video resolution, their approaches and outcomes are vastly different.
AI-powered video upscaling has revolutionized how resolution is enhanced, delivering far better results than traditional upscaling albeit with higher demands. As AI becomes more accessible and efficient, it is likely to become the standard for video enhancement across all platforms.
As the demand for high-quality digital media continues to grow, AI-powered video upscaling is expected to evolve rapidly. Several future trends are shaping the next generation of video enhancement technologies.
Thanks to advancements in edge computing and optimized neural networks, real-time AI upscaling will likely be performed directly on smartphones, TVs, and gaming consoles. Consequently, users will enjoy improved visuals without relying on cloud-based processing or external software.
With the rise of AR and VR applications, clear and immersive visuals are critical. AI video enhancement will be used to upscale 2D and 3D assets in real-time, providing smoother, more lifelike environments for education, training, and entertainment.
Future systems may allow users to select or train AI models based on personal preferences or content types. For example, one model could be tailored for anime upscaling, while another enhances historical footage with colorization. As a result, content becomes both clearer and stylistically consistent.
Combining traditional interpolation methods with AI-powered techniques may lead to more efficient upscaling solutions. These hybrid systems could reduce processing time while maintaining visual fidelity, making high-quality video more accessible.
AI algorithms will continue to be optimized for energy efficiency. Reduced power consumption will allow high-quality upscaling on battery-powered devices without compromising performance, benefiting both mobile users and the environment.
A single AI model capable of upscaling videos across multiple platforms (TVs, mobile apps, browsers, and VR headsets) may be introduced. This universal approach would streamline workflows and offer consistent video quality regardless of the device.
As AI tools become more powerful, so does the potential for misuse. The industry is expected to implement verification and watermarking systems that ensure enhanced videos remain authentic and unmanipulated for ethical use.
The future of AI-powered video upscaling is both exciting and expansive. With ongoing innovation, the boundaries between low-resolution and high-quality video will continue to fade, transforming how we consume and interact with digital media.
AI-powered video upscaling has emerged as a game-changing technology, offering unprecedented opportunities to enhance video quality across various industries. From film restoration to gaming, security, and content creation, the ability to upscale videos with AI ensures that even low-resolution footage can be transformed into visually stunning media, suitable for modern high-definition displays.
Despite some challenges such as high computational demands and limitations with severely degraded footage the advantages of AI upscaling far outweigh the drawbacks. With benefits including improved visual quality, cost-effective content upgrades, and real-time processing, it is no wonder that AI-powered video upscaling has gained widespread adoption.
As this technology continues to evolve, future trends will likely bring even more powerful and efficient solutions. Real-time upscaling, integration with AR/VR, and personalized models are just the beginning. With these advancements, AI-powered video upscaling will only become more accessible and versatile, driving improvements in both user experience and production efficiency.
© 2025 LeyLine