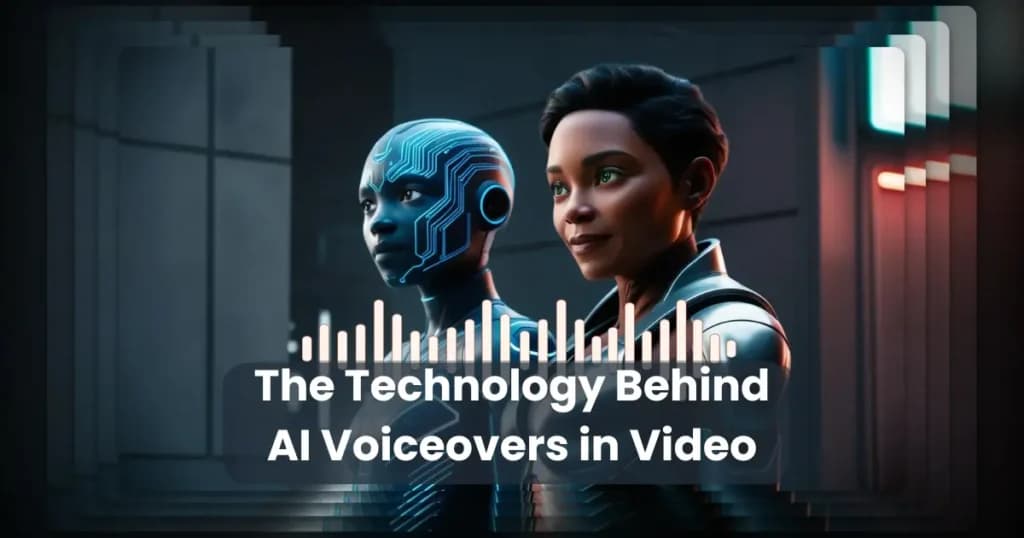
Contents
In today’s fast-evolving digital landscape, understanding how image-to-video AI works explained is essential for anyone involved in content creation, machine learning, or visual storytelling. This technology enables a single image to come alive through motion, making it an exciting development in the field of artificial intelligence.
Moreover, by leveraging deep learning, neural networks, and motion prediction, image-to-video AI goes far beyond traditional animation techniques. Rather than requiring frame-by-frame input, these models analyze visual data, infer movement, and synthesize video in a highly efficient and visually coherent manner.
As a result, this innovation is reshaping industries—from advertising and entertainment to education and augmented reality. In the following sections, we will explore its key components, step-by-step functionality, real-world use cases, and what the future holds for this transformative AI.
At its core, image-to-video AI is a technology that transforms still images into dynamic video sequences using artificial intelligence. Instead of relying on manual animation or multiple input frames, this technique generates motion from a single image by predicting how elements within the image would logically move over time.
More importantly, this process is made possible by deep learning models trained on vast datasets of videos and images. These models learn patterns of motion—such as how a person’s face might shift expressions or how wind might move through trees—and then apply those learned patterns to static input.
Unlike traditional animation tools, which require extensive manual input, image-to-video AI automates the animation process. Consequently, it saves time, reduces production costs, and opens creative possibilities for those without technical animation skills. Whether you’re working in media, marketing, or research, the ability to animate from a photo with such precision and realism is a game-changer.
To fully grasp how image-to-video AI works explained, it’s essential to explore the foundational technologies that drive it. These technologies work together to analyze static images, simulate motion, and generate video frames with realism and fluidity.
First and foremost, neural networks—particularly convolutional neural networks (CNNs)—are used to extract meaningful features from input images. These networks identify patterns such as shapes, textures, and facial landmarks. Once extracted, these features are passed along to models responsible for motion prediction and video generation.
In addition, generative adversarial networks (GANs) play a critical role in creating believable motion. A GAN consists of two models: a generator and a discriminator. While the generator attempts to create realistic video frames, the discriminator evaluates them against real video data. Through constant feedback, both models improve, resulting in high-quality animated sequences that appear lifelike.
Next, optical flow algorithms estimate motion between pixels in an image over time. These algorithms are especially useful for determining how objects or facial features might naturally move. By applying optical flow techniques, AI models can create smooth transitions and motion continuity, even when starting from a single frame.
Furthermore, pose estimation is used when animating human or animal subjects. Keypoints such as eyes, joints, or facial features are detected and tracked. These serve as anchors for generating motion, ensuring that the animated movements follow logical and anatomically accurate paths.
Finally, ensuring temporal coherence is crucial. Without it, the video output may appear jittery or inconsistent. Techniques are implemented to verify that each generated frame aligns smoothly with the previous one, maintaining a realistic sense of time progression.
Together, these technologies form the backbone of modern image-to-video AI systems. They work in harmony to bring still images to life—accurately, efficiently, and creatively.
Now that the core technologies have been covered, let’s break down how image-to-video AI works explained in a step-by-step process. Each stage is designed to convert a static image into a coherent and visually dynamic video using learned motion patterns and advanced neural computations.
To begin with, the input image is preprocessed to ensure clarity and optimal quality. Any noise or distortion is removed, and the image is enhanced through filters and normalization techniques. This preparation stage is vital because clean, high-resolution input leads to more accurate feature detection later on.
Next, deep learning models—specifically CNNs—analyze the image to detect features such as contours, facial landmarks, textures, and objects. These features serve as the blueprint for animating the image and predicting how elements might move.
Then, the extracted features are used by a generator (usually within a GAN) to synthesize intermediate frames. Rather than producing random visuals, these frames follow plausible motion patterns, giving the illusion of natural movement from one moment to the next.
At this point, motion is introduced through the integration of pose estimations or optical flow predictions. For example, if the input is a human face, AI can infer blinking, head tilting, or lip movement. This synthesized motion is applied frame by frame to bring the static image to life.
After generating motion, ensuring consistency across frames becomes essential. Temporal coherence techniques are applied to eliminate any sudden jumps or inconsistencies. This guarantees that the resulting video feels fluid and realistic rather than fragmented or artificial.
Finally, the AI fine-tunes the entire sequence. This includes adjusting lighting, shadow continuity, and texture stability to match real-world video standards. The final output is then rendered into a smooth video clip, ready for use across various platforms.
By following this structured pipeline, the AI system transforms still images into convincing videos. Not only is the process efficient, but it also delivers impressive results that continue to improve as models evolve.
Now that how image-to-video AI works explained has been broken down step by step, it’s time to explore where this technology is actually being used. Far from being a futuristic concept, image-to-video AI is already transforming a wide range of industries with practical, scalable applications.
To start, the film and gaming industries are major adopters of this technology. By animating concept art or character portraits, studios can rapidly prototype scenes or generate promotional content without shooting additional footage. Moreover, older video content can be revitalized by animating static imagery from archives.
Additionally, marketers use image-to-video AI to create personalized, animated content for social media, email campaigns, and product demos. Instead of hiring a video crew, brands can now animate a single product photo into a short video—cutting costs and turnaround time dramatically.
In education, static textbook diagrams and illustrations can be turned into motion-based visual aids. This not only enhances comprehension but also makes learning more interactive and engaging for students of all ages.
Furthermore, social media platforms and AR applications use this technology for facial animations and motion effects. For instance, a selfie can be animated to blink, smile, or speak—enhancing user engagement through creative, shareable content.
In the healthcare sector, AI-generated video simulations are being used to visualize body parts or procedures from a single medical image. This improves diagnostics, training, and patient communication without the need for costly 3D scanning.
Finally, museums and digital historians employ image-to-video AI to animate historical figures or artworks. As a result, visitors can experience an interactive journey through time, where ancient portraits seem to come to life.
These real-world applications prove that image-to-video AI is not just a novelty—it’s a powerful tool with wide-reaching implications across creative, educational, and scientific fields.
While understanding how image-to-video AI works explained offers exciting insight into its mechanics, it’s equally important to examine its strengths and weaknesses. This balanced view helps users and developers determine where this technology fits best—and where caution should be applied.
1. Time Efficiency
First and foremost, one of the greatest advantages of image-to-video AI is its speed. A single image can be transformed into a short video within minutes, removing the need for lengthy manual animation processes.
2. Cost-Effectiveness
Additionally, this technology significantly reduces production costs. With fewer tools, fewer personnel, and minimal hardware requirements, small businesses and individual creators gain access to high-quality animation at a fraction of the usual expense.
3. Accessibility
Moreover, no advanced design or animation expertise is needed. This opens up creative possibilities for marketers, educators, and social media users who may not have technical backgrounds.
4. Enhanced Creativity
Because motion is generated automatically, creators can experiment more freely without being limited by time or skill constraints. The AI handles the complexity, allowing more focus on storytelling and aesthetics.
5. Wide Versatility
Finally, this technology adapts to diverse use cases—from entertainment and education to marketing and virtual reality—making it a versatile tool across industries.
1. Motion Accuracy Issues
However, despite its strengths, image-to-video AI can sometimes produce unnatural or incorrect movements—especially when input images are complex or poorly structured. Facial distortions and glitchy animations may occur.
2. Dependency on Training Data
Another limitation lies in data dependency. If the AI model hasn’t been trained on diverse motion datasets, its output will lack variation and realism.
3. Ethical Concerns
Furthermore, this technology can be misused for deepfakes or misleading visual content. Without clear regulation or watermarking, the authenticity of animated videos can become questionable.
4. Lack of Emotional Subtlety
In some cases, AI-generated videos may miss the finer emotional cues found in human animation. The results may feel flat or robotic when compared to professionally animated content.
5. Limited Real-Time Use
Lastly, while processing speed is fast, real-time generation is still a challenge for many systems. Live applications—such as video conferencing or gaming—may not fully benefit from this technology just yet.
Although image-to-video AI brings powerful advantages, it must be used thoughtfully and with awareness of its boundaries. As the technology continues to mature, many of these limitations are expected to diminish—making future applications even more promising.
Looking ahead, the potential of image-to-video AI continues to grow at a rapid pace. As researchers and developers push the boundaries, many exciting improvements are already emerging—making the future of this technology more dynamic and impactful than ever.
To begin with, one of the most anticipated advancements is real-time processing. Currently, most systems require a few minutes or more to render outputs. However, with hardware acceleration and optimized algorithms, instant video generation from static images will soon become feasible, especially for live-streaming, gaming, and AR experiences.
Additionally, future AI models will be better equipped to interpret and replicate subtle emotional expressions. Improved facial mapping and emotional intelligence will allow videos to feel more lifelike, thereby bridging the gap between synthetic and human-like communication.
In the coming years, more intuitive tools will give users finer control over the animation process. Rather than relying solely on automated output, creators will be able to specify emotions, gestures, or camera angles using simple sliders, prompts, or even voice commands.
Moreover, image-to-video AI will be seamlessly integrated with AI-powered voice synthesis, language translation, and scriptwriting tools. As a result, entire scenes—including dialogue, motion, and background—could be generated from a single image and a text prompt.
At the same time, developers are working on advanced watermarking systems and deepfake detection tools. These will help prevent misuse and ensure that AI-generated videos remain traceable and ethically transparent, which is especially critical in journalism and education.
Finally, as adoption spreads, industry-specific versions of image-to-video AI will emerge. For instance, healthcare tools will focus on patient simulations, while entertainment platforms might offer high-fidelity avatar animation engines for creators.
In summary, the future of how image-to-video AI works explained points to broader accessibility, deeper realism, and stronger ethical grounding. As innovation continues, this technology will become not only more powerful—but also more responsibly integrated into our daily lives.
In conclusion, how image-to-video AI works explained reveals an extraordinary blend of deep learning, neural networks, and motion prediction that transforms static images into dynamic videos. From preprocessing and feature extraction to motion synthesis and output rendering, every step plays a crucial role in ensuring fluid and believable results.
Throughout this article, it has been shown that the technology is already revolutionizing fields such as entertainment, education, healthcare, and digital marketing. While current limitations—like motion inconsistencies or ethical risks—should not be overlooked, ongoing advancements are expected to address many of these concerns.
Moreover, the future holds incredible promise. Real-time generation, emotional realism, and ethical AI governance are just a few of the innovations on the horizon. With these in place, the utility and trustworthiness of image-to-video AI will only strengthen over time.
Ultimately, how image-to-video AI works explained is not just about the mechanics—it’s about unlocking new creative potential and redefining how we interact with visual media in the digital age.
© 2024 LeyLine