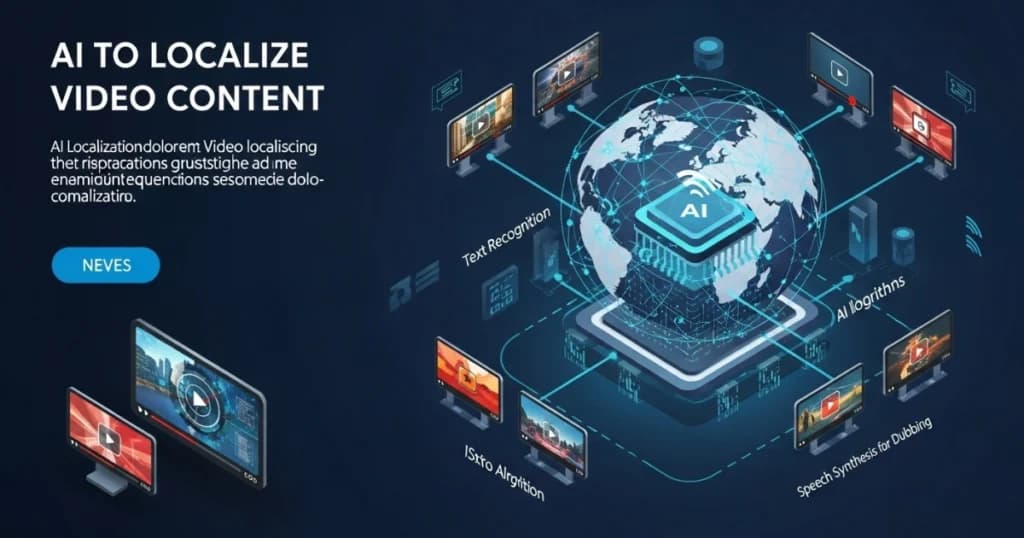
Contents
In the digital age, the role of machine learning in AI video creation has become increasingly significant. As demand for video content grows across industries, creators are turning to machine learning to automate, enhance, and accelerate the production process. By training algorithms to analyze vast datasets, machine learning empowers AI tools to generate video content that is realistic, engaging, and highly tailored to specific audiences.
Moreover, with the rise of platforms that prioritize video such as YouTube, TikTok, and Instagram there’s a pressing need to produce high-quality visual content quickly and cost-effectively. Machine learning bridges this gap, transforming traditional video creation workflows and enabling entirely new forms of visual storytelling. In the following sections, we’ll explore how this technology works, its real-world applications, and why it’s shaping the future of media.
Machine learning is a branch of artificial intelligence that allows computers to learn and make decisions from data without being explicitly programmed. Instead of following a fixed set of rules, machine learning systems identify patterns, adapt to new information, and improve their performance over time. This makes them incredibly powerful for tasks that involve large-scale data analysis, prediction, and automation.
In the context of AI video creation, machine learning enables systems to understand visual elements, recognize speech patterns, generate lifelike animations, and even create entire video scenes from text prompts. These capabilities are made possible by training algorithms on massive datasets that include video footage, images, audio clips, and text. As the system processes more data, it becomes better at mimicking real-world visuals and audio, enhancing the role of machine learning in AI video creation dramatically.
Ultimately, machine learning acts as the intelligence behind the automation, learning how to think like a director, editor, or animator just at machine speed and scale.
Machine learning serves as the engine that drives AI video creation. By analyzing enormous amounts of video data, these algorithms learn how scenes are structured, how humans move and speak, and how visual storytelling unfolds. This deep understanding allows machine learning models to generate, modify, and optimize video content with minimal human input.
To begin with, machine learning automates tedious processes like scene detection, object recognition, and facial tracking. These tasks, once requiring hours of manual editing, can now be completed in seconds. Moreover, text-to-video systems use natural language processing—another branch of machine learning to transform scripts into animated visuals or realistic scenes. This process is revolutionizing content creation across entertainment, marketing, and education.
Another important aspect is voice synthesis. Machine learning algorithms can clone voices, match lip-sync to speech, and adjust tone based on context. This enables creators to produce multilingual or personalized content without recording new audio each time.
Furthermore, machine learning models continuously refine their output. With each new video generated, the system analyzes viewer feedback, learns what works, and applies that knowledge to improve future content. This feedback loop is central to enhancing the role of machine learning in AI video creation making it smarter, faster, and more creative over time.
By streamlining every phase of production from scripting to rendering machine learning is not just assisting creators; it’s becoming an indispensable creative partner.
To fully understand the role of machine learning in AI video creation, it’s essential to explore the specific models that make this technology so effective. Each model serves a unique purpose, working together to automate and enhance different aspects of video production.
GANs are perhaps the most revolutionary machine learning models in video creation. They consist of two neural networks the generator and the discriminator that compete with each other. The generator creates synthetic content, while the discriminator evaluates its authenticity. Over time, this competition results in hyper-realistic visuals, from human faces to entire backgrounds, perfect for AI-generated video scenes.
CNNs are particularly adept at analyzing visual data. In AI video creation, they help identify and process objects, patterns, and movements within video frames. They’re commonly used in tasks like facial recognition, scene segmentation, and visual effects automation.
RNNs are designed to handle sequential data, which makes them ideal for video processing where time and sequence matter. They predict the next frame in a video or synchronize motion with audio, playing a key role in smooth transitions and animated actions.
Originally developed for language processing, transformer models have found a strong foothold in video creation. They help AI understand context across long video sequences, improving everything from voiceover timing to narrative coherence. Transformers also power tools like script-to-video generators, converting written content into visual scenes.
Autoencoders compress and then reconstruct data, making them useful for video compression and enhancement. They help maintain high video quality while reducing file size and optimizing performance, especially for streaming or mobile use.
Each of these models plays a critical part in shaping the role of machine learning in AI video creation. When combined, they enable systems to mimic the decisions of human editors, storytellers, and animators with remarkable accuracy and efficiency.
The rapid integration of machine learning into digital media has transformed how videos are produced, edited, and consumed. Let’s take a closer look at the key benefits that highlight the role of machine learning in AI video creation.
Machine learning dramatically shortens production timelines. Tasks like scene detection, object tracking, and audio syncing, which once required hours of manual labor, are now completed in seconds. This allows creators to generate high-quality videos at scale with minimal effort.
By automating large portions of the workflow, machine learning reduces the need for extensive human resources. There’s less dependence on expensive software, voice actors, or production teams making professional-grade video creation accessible even to small businesses and solo creators.
Machine learning algorithms can analyze user data and adapt content accordingly. Whether it’s customizing a video’s language, visuals, or tone, AI can create highly tailored experiences that resonate with specific audiences. This personalization leads to greater engagement and retention.
With tools powered by machine learning, creators can experiment with visual styles, deepfakes, or even generate entire animated scenes from scratch. These capabilities expand creative boundaries and enable storytelling that was previously impossible without massive budgets.
Machine learning models continuously learn from viewer behavior. This means content can be adjusted in real time to improve performance—whether by changing thumbnails, reordering scenes, or refining dialogue delivery for clarity.
Perhaps the most compelling benefit is scalability. From producing hundreds of personalized ads to generating entire video libraries for e-learning platforms, machine learning makes it possible to replicate and modify content at unprecedented levels.
In short, the role of machine learning in AI video creation goes beyond automation. It empowers creators to be faster, more innovative, and more responsive to audience needs paving the way for a new era of digital storytelling.
Understanding the role of machine learning in AI video creation becomes even clearer when we examine how it’s being used in real-world settings. From entertainment to education, machine learning is driving innovation across multiple industries.
Major film studios use machine learning to streamline post-production workflows. Tasks like rotoscoping, color grading, and CGI rendering are enhanced by AI, cutting costs and saving time. AI-generated characters and backgrounds are also being used to fill in scenes, reducing reliance on physical sets or extras.
Platforms like TikTok, Instagram, and YouTube have embraced AI tools that allow creators to auto-edit videos, apply filters, or generate content based on trends. Machine learning recommends edits, music, and hashtags to improve reach and engagement demonstrating its core impact on fast-paced content creation.
Educational platforms use AI video creation tools to develop personalized learning modules. Machine learning can turn text lessons into animated explainers or simulations, adapting the tone and pace to individual learning styles. This has transformed how knowledge is delivered, especially in remote learning environments.
Brands utilize machine learning to create hundreds of video ad variations tailored to different demographics, languages, and viewer behaviors. AI-driven video creation allows for fast A/B testing and optimization, helping businesses improve ROI without hiring large creative teams.
In video games and virtual environments, machine learning helps generate realistic cutscenes, dynamic character interactions, and auto-generated storylines. This creates immersive narratives that evolve based on player choices, all while reducing production bottlenecks.
Newsrooms are now using AI to automatically generate video summaries of articles, interviews, or press releases. These quick, engaging clips boost audience retention and make information more digestible on mobile platforms.
In each of these domains, the role of machine learning in AI video creation is to simplify complex tasks, reduce manual intervention, and enhance the creative potential of human teams. As the technology matures, its impact will only grow deeper and broader.
While the role of machine learning in AI video creation offers transformative benefits, it also presents several challenges and limitations that must be carefully managed. Understanding these issues is crucial for responsible development and deployment.
Machine learning models are only as good as the data they are trained on. If the training data is biased, low-quality, or unrepresentative, the output will reflect those flaws. In AI video creation, this can result in distorted visuals, mismatched audio, or inaccurate representations that damage credibility and viewer trust.
One of the most controversial outcomes of machine learning in video is the rise of deepfakes. While they can be used for satire or creative purposes, they also raise serious ethical concerns around misinformation, impersonation, and consent. Safeguards and verification tools must evolve to address these threats.
Relying too heavily on machine learning tools can lead to a creative crutch. As AI takes over repetitive tasks, there’s a risk that creators may lose hands-on skills or fall into formulaic content generation, stifling originality.
Training and running machine learning models require significant computing power, energy, and storage. This can make AI video creation expensive or unsustainable for smaller teams, especially when dealing with high-resolution or long-form content.
AI-generated videos often use data from existing media to train models, raising questions about ownership, copyright infringement, and fair use. As machine learning systems become more autonomous, legal frameworks must catch up to define who owns the content created by AI.
While machine learning has made impressive strides, it still struggles with context, nuance, and cultural sensitivity. Videos generated by AI may include unintended messaging or miss emotional cues that human creators instinctively understand.
Despite these challenges, continuous innovation and ethical guidelines are helping to shape a more secure and responsible future. By addressing these limitations, we can strengthen the role of machine learning in AI video creation and maximize its potential across industries.
As technology continues to evolve at a rapid pace, the role of machine learning in AI video creation is set to expand dramatically. The coming years will bring not only improved tools but also entirely new ways of thinking about video production, storytelling, and audience engagement.
Future machine learning models will be able to generate ultra-realistic videos that are nearly indistinguishable from those filmed with cameras. From facial expressions to natural lighting and shadow effects, AI will soon replicate real-world physics with unprecedented accuracy.
We’re moving toward real-time video creation where AI can generate and edit content on the fly. This will revolutionize industries like live broadcasting, gaming, and virtual events, enabling creators to adapt content instantly based on viewer interaction and feedback.
Future AI will harness deeper user data to craft hyper-personalized video experiences. Imagine training videos that adjust tone and complexity based on user behavior or marketing videos that adapt visuals in real time depending on viewer preferences.
As tools become more intuitive and affordable, machine learning will empower non-experts to produce studio-quality videos. This democratization will unleash a new wave of creativity and allow diverse voices to shine without technical barriers.
Machine learning will play a major role in blending video with immersive technologies like AR and VR. AI-generated 3D environments, interactive video narratives, and spatially-aware storytelling will redefine how we consume visual media.
With growing concerns around deepfakes and misinformation, the future will demand transparent AI models and better verification tools. Machine learning systems will include built-in checks to ensure ethical use and prevent misuse of AI-generated content.
The future is not just about automation—it’s about collaboration. Human creativity, guided and empowered by intelligent systems, will define the next generation of content. As machine learning grows more sophisticated, so too will its role in AI video creation, transforming both how we make videos and how we experience them.
In today’s digital landscape, the role of machine learning in AI video creation is no longer a futuristic concept—it’s a present-day revolution. From speeding up production to enabling personalized content and reducing costs, machine learning is redefining the boundaries of what’s possible in video creation.
As we’ve explored, machine learning powers everything from deep learning-based video synthesis to real-time editing and animation. It empowers creators to tell richer stories, businesses to scale faster, and audiences to engage more deeply with content tailored just for them. However, with great power comes great responsibility. Ethical challenges, legal concerns, and creative limitations must be addressed to ensure the technology is used wisely.
Looking ahead, machine learning will not only enhance current workflows but also unlock entirely new creative possibilities through real-time generation, hyper-personalization, and immersive AR/VR experiences. As AI continues to evolve, so will its capacity to support and amplify human creativity.
In summary, the role of machine learning in AI video creation is both transformative and indispensable. Those who embrace it now are not just adapting to change they’re leading it.
© 2025 LeyLine